What do we understand as bias? Can bias be good or destructive in everyday human interaction? Is bias in humans different from bias in AI? There are a number of questions associated with bias in the AI industry. As businesses deploy AI solutions, care must be taken to avoid setting up models that come off as discriminatory to both consumers and regulators.
However, solving the problem of bias is usually not that simple. With businesses having requirements like ‘company culture’ fit as an employment criterion, how does an algorithmic model decide that a job applicant is fit? The way such an applicant talks? Keywords used in the CV? A human HR agent may easily detect if an applicant is culturally fit for the company after having a 3-minute chat. Can an AI HR software easily do this?
In most recruiting algorithms, the software examines the CV of applicants based on keywords. Could this lead to instances where an applicant is tagged culturally unfit, because of the absence of a keyword? As we will see, this is possible but then how do businesses deploying AI reduce bias in their models? First, we must get the basics.
How does bias in humans translate into bias in AI models?
Similar to the way neurons in our brains work to build reinforcement learning, neural networks are used by AI to build solutions enabled with reinforcement learning. Is bias in humans normal? Some may argue that bias is an integral part of human interaction. Humans interact with other humans and objects every day based on bias. That this happens does not necessarily endorse the negative outcomes of bias, such as discrimination. Algorithms are designed by humans to solve human problems and in understanding these problems, bias may gradually creep in.
How about bias in AI? Some define bias in AI as a deviation from the truth in data collection and data analysis. Bias may creep into your model where the dataset is not representative enough, or where data is inaccurate or unstructured. The outcome of bias makes it a concrete precondition to consider when creating AI models. Outcomes such as disenfranchisement, discrimination based on sex, age, etc. are negative. Where outcomes are negative, it can also be costly to the reputation of business.
Corporations such as Amazon and Facebook have come under fire for deploying discriminatory software. In 2018, Reuters reported that Amazon’s recruiting algorithm had been discriminating against women. In a project later shutdown, the Amazon algorithm was trained with about 10 years of data (sample resumes) which was male-dominated, resulting in an outcome not contemplated. Asides its reputational costs, regulators frown against discriminatory models, putting liable businesses at risk of regulatory fines and sanctions.
The role of data in AI Bias
Data is central to how an AI functions. Training data is cited as one of the ways bias can creep into algorithms. Where the trained data is built from biased human decisions, or retains certain structural inequalities, the risk to creating bias is higher.
However, this also means that we find the solutions in the problems. Where data is consistent, well structured and representative, bias is reduced in the outcomes of the models.
Some techniques have been highlighted as necessary for building bias-proof solutions.
- Understand what problem you want to solve: sometimes, bias creeps in when a problem is being framed. Building data structures on such problems, bias in the outcomes is unavoidable. Therefore, production teams should carefully frame problems in a manner which does not trigger bias in outcomes.
- How you collect the data: as a business, you need to verify that datasets are representative of a problem you seek to solve. Where the data is unrepresentative, bias becomes more likely. For example, the Amazon team might have gathered a more representative dataset to train and test the model.
- Preparing the data: this goes beyond collecting data. In cleaning, training and labeling a dataset, the engineering team has to verify whether variables selected are likely to encourage bias. Some flagged variables include gender, age, etc. In Amazon’s case, this implies it may have turned off the gender variable in its dataset before training the model.
Engineering teams building AI solutions should understand the best practices that ensure the quality of data. Some of these best practices include properly labeling data, reducing noise and keeping up-to-date datasets.
Alternatively, AI engineering teams can rely on efficient data collectors. The process of quality and efficient data collection is a continuous one, requiring recurrent tests. Therefore, if you are a team leader, you may want to ask the following questions to test the resilience of your datasets:
- Is the data complete relative to the target market?
- Are there any unusual trends from the dataset from specific codes such as gender, race, etc.?
- Are there any gaps in the data collection process?
- Can more data be obtained?
Other simple Strategies for preventing bias in your AI model:
a. Outsource data collection:
Strategists have observed that data collection is one of the toughest aspects of building AI solutions. Data scientists also confirm that a bulk of the time is spent on preparing data. This takes a great toll on the resources of small businesses that are deploying AI solutions with small engineering teams.
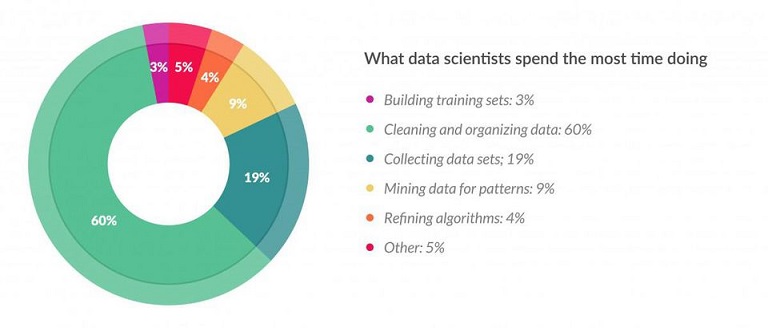
Businesses have a bigger advantage where they outsource their data collection tasks to experienced teams. Companies like Datix AI provide data solutions cleaned and trained for the purpose of algorithms built for solving problems. With a dedicated team of data scientists and analysts, Datix AI provides a variety of datasets including text, images, audio, video. With access to crowdsourced data, representation is hardly a problem with data sourced from Datix AI.
b. More representative engineering teams:
Another observation made by experts on the subject of bias is that engineering teams need more representative participation. All algorithms are built by humans to solve human problems. While we cannot eliminate bias as a behavioral instinct, it may prove destructive to build these biases into algorithms.
As they are applied in society, AI models should contribute more to our growth. A simple way for this to be done is to open up engineering teams to more representative views based on gender, race, etc. As representation becomes more equitable, solutions provided using AI become less susceptible to bias.
c. Keep learning
The iteration process should not only stop with the AI model. As we explore the yet unfamiliar world of AI, the learning must not stop. As humans are bound by their perception, iteration keeps us questioning some things we believe as we interact from day to day. It is therefore essential to form a learning culture as we build better AI solutions.
Providing quality datasets is beyond using public databases, Datix AI builds data solutions you need to train your AI models. We provide services such as providing datasets for your AI model, introducing your business to machine learning best practices, and service different technology innovators and builders. Contact Us (insert hyperlink) today to learn more about creating clean datasets for your model.